The World’s Most Sensitive Experiment Is Controlled by AI
Large, complex experiments are searching for “dark matter”. An Austrian research team has now shown how AI can help.
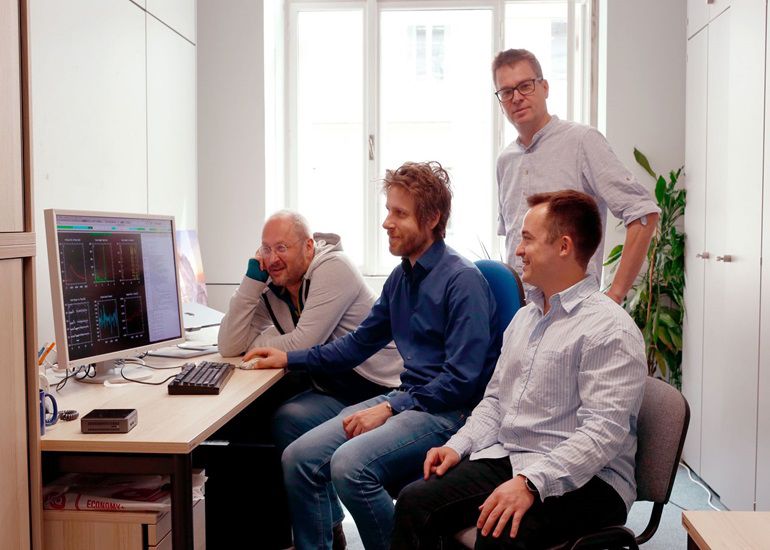
The search for new particles often requires sensitive detectors and these in turn require sensitive calibration of their control parameters. In order to make this calibration more efficient in the future, researchers from HEPHY Vienna and TU Wien have used an AI system to calibrate detectors from the CRESST experiment - a groundbreaking step for future experiments. This project has now been published in the journal “Computing and Software for Big Science”.
One of the biggest unanswered questions in modern physics is the nature of dark matter. There is irrefutable evidence that this type of matter, which has not yet been directly measured, must exist, and numerous experiments are being carried out in the search for it. “If dark matter is a particle, which we assume it is, then there is a dark matter particle on Earth with a volume roughly the size of a coffee cup,” explains Jochen Schick, Head of the Institute for High Energy Physics (HEPHY) and professor at the Vienna University of Technology.
Measuring the interaction with such a particle is the aim of the CRESST experiment, in which highly sensitive detectors are operated in a laboratory that is extremely well shielded against background radiation: the Laboratori Nazionali del Gran Sasso, about a two-hour bus ride east of Rome.
Ice-cold Crystals and Fractions of Brain Waves
Such a detector consists of a crystal onto which a metal film is vapor-deposited. The metal, tungsten, a so-called superconductor, changes its electrical resistance very suddenly at a certain, very low temperature. This temperature is around 10 millikelvin or -273.14 degrees Celsius, i.e. just above absolute zero.
When the system is cooled to exactly this temperature, a single collision of a dark matter particle triggers enough vibrations in the crystal lattice to slightly heat the metal film and drastically change its electrical resistance. This in turn generates an electronic signal that can be measured. “With this technology, we can resolve energies of single electron volts. That’s about a billionth of the energy a brain cell needs to fire,” explains Florian Reindl, assistant professor at TU Wien.
Self-learning Control Enables Larger Experiments
The calibration of such detectors, namely the equilibrium temperature and current, is therefore delicate. This task was previously carried out for the CRESST experiment using a semi-automatic method that requires individual adjustment for each detector. “Each detector is an individual, so to speak,” says Felix Wagner, who recently completed his doctorate at HEPHY and TU Wien. “We usually reserve one afternoon per detector for this task, but it often takes even longer.”
This amount of time is manageable for smaller experiments, but problematic for future experiments in which hundreds of these detectors are to be operated. Artificial intelligence, especially reinforcement learning, can learn the behavior of a system live while it is being controlled. Wolfgang Waltenberger, Senior Scientist at HEPHY, makes a comparison: “It’s like a person driving a new car. Every car reacts a little differently, and during the test drive the driver learns how to deal with the vehicle’s peculiarities in the best possible way.”
In February 2023, the researchers tested their method in a dedicated time window on the CRESST experiment. The AI was able to calibrate all the detectors on which it was tested within around 90 minutes with satisfactory results. “These results are a proof-of-principle that our method is excellently suited to take on future, large experimental setups,” says Wagner happily. “This allows us to achieve scalability without any loss of quality.”
Autonomous Experiments in the Future?
The research project was a collaboration with the Center for Artificial Intelligence and Machine Learning (CAIML) and the Machine Learning Research Unit of the Faculty of Computer Science at TU Wien. “It is to be expected that reinforcement learning will increasingly be used for the autonomous control of devices in the future. Among other things, this is interesting for the automation of experiments in order to advance researchers in their work even more efficiently, as has been done here,” explains Clemens Heitzinger, Professor at TU Wien and Head of CAIML.
The calibration method with reinforcement learning is to be integrated as a fixed component in the control system of the CRESST experiment as part of a hardware upgrade planned for 2025. The electronics for this upgrade will be manufactured at HEPHY.
Original Publication
German Version of the Article
The German version of this article was published on the website of TU Wien.