Johannes Fürnkranz: “Towards Deep and Interpretable Rule Learning”
CAIML Seminar with Johannes Fürnkranz.
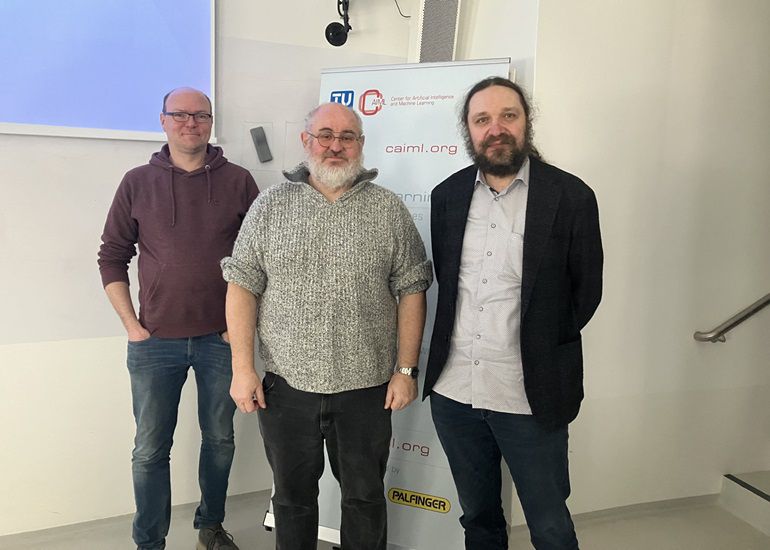
On March 15, 2024, the CAIML seminar with Johannes Fürnkranz took place.
Abstract
Inductive rule learning is concerned with the learning of classification rules from data. Learned rules are inherently interpretable and easy to implement, so they are very suitable for formulating learned models in many domains. Nevertheless, current rule learning algorithms have several shortcomings. First, with respect to the current praxis of equating high interpretability with low complexity, we argue that while shorter rules are important for discrimination, longer rules are often more interpretable than shorter rules, and that the tendency of current rule learning algorithms to strive for short and concise rules should be replaced with alternative methods that allow for longer concept descriptions. Second, we think that the main impediment of current rule learning algorithms is that they are not able to learn deeply structured rule sets, unlike the successful deep learning techniques. Both points are currently under investigation in our group, and we will show some results.
About the Speaker
Johannes Fürnkranz is an Austrian mathematician, computer scientist, and AI researcher, since 2019 affiliated with the Institute for Application Oriented Knowledge Processing, Johannes Kepler University Linz, previously professor in knowledge engineering at the TU Darmstadt. He holds a Ph.D. in 1997 from Vienna University of Technology under advisor Gerhard Widmer. His research spans various AI-fields, such as machine learning, data mining, and more recently Monte-Carlo tree search. He is editorial board member of various scientific AI-Periodical, such as the Journal of Artificial Intelligence Research (JAIR), and is editor-in-chief of the bimonthly Data Mining and Knowledge Discovery journal.
Slides
Here you can download the slides:
Download the slides.